From BI to AI: Reporting vs. Solving Problems
- info0769516
- Feb 12
- 4 min read
The Purpose of Analytics
NewVantage Partners found some time ago that over 9 in 10 organisations have achieved measurable value from data and analytics investments. However, what does “value” really mean? It can range from routine reporting to uncovering insights, identifying solutions, and at the other end of the scale, driving innovation. Most organisations are now capable of collecting more data than they can make sense of - so how can they best find the signal in the noise?
Routine reporting is important, but organisations should be mindful that their analytics teams do not get trapped in a cycle of Business as Usual (BAU) reporting, which limits their ability to provide more strategic value. A focus shift from reporting to problem solving can harness more value from data - from descriptions to predictions and prescriptions. From reactive to proactive.
The Difference Between Reporting and Problem Solving
Reporting and problem solving represent two distinct approaches to working with data. Reporting is often answering the question, “What happened?” This is typically achieved through standardised, descriptive analytics that monitor business processes. While useful for maintaining operational awareness, reporting can sometimes lack the depth or detail needed to solve problems.
Problem solving involves digging deeper into data to uncover patterns, predict what will happen and propose actionable solutions that can be scaled. It moves from addressing questions like “Why did this happen?” to “What should we do next?”.
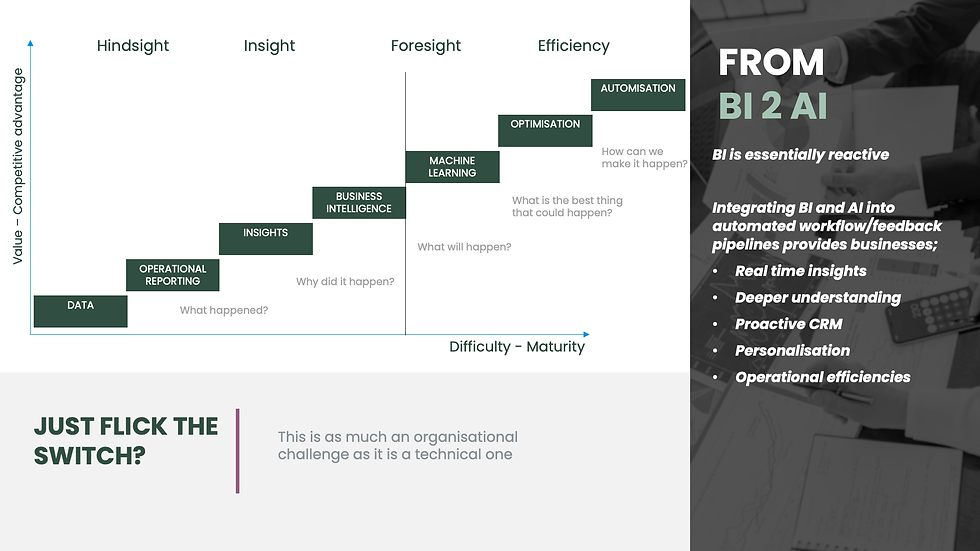
Overcoming The Transition Barrier
It’s not always a straight path from A to B. Shifting mindset from reporting to problem solving may involve an initial “productivity shock”. This organisational transition is often uncomfortable for some people, leading to resistance and productivity loss.
Few question the benefits advanced analytics can bring. However, many lack a clear implementation vision across their people, platform and processes. It’s often not clear what should be the first step and the most effective sequence of steps after that. Rome was not built in a day - big change requires milestone wins along the journey to build and maintain momentum.
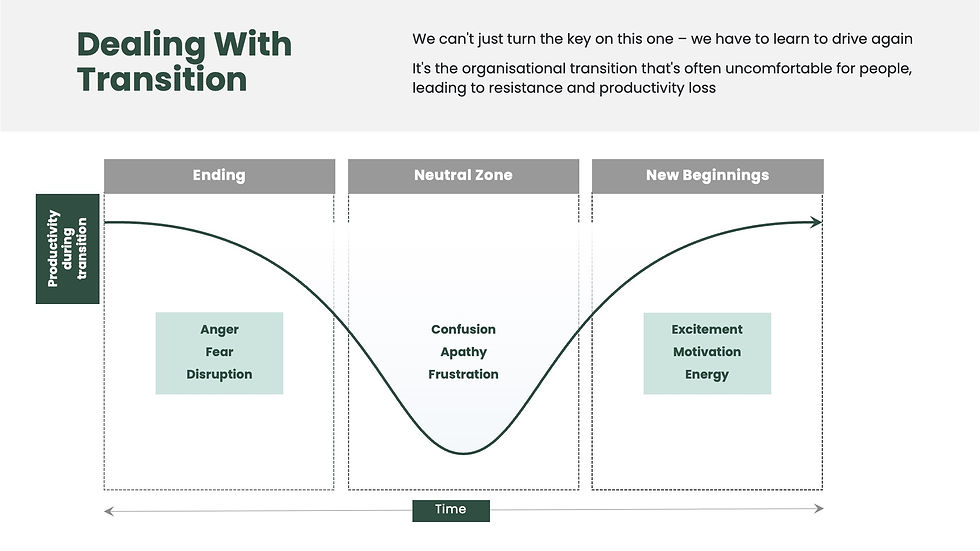
Empowering Analytics Teams
Analytics teams can only drive improvement if they are aligned with business goals. Defining clear objectives and encouraging dialogue between the business focused and data focused people can often be the first step - they need to share the same language and vision.
Leadership buy in is essential in this transformation. Leaders must advocate for analytics driven decision making and invest the appropriate amount of resourcing and time.
A Deloitte survey of Chief Data Officers' top 3 priorities for 2023 and beyond found 68% wanted to improve the way they used data and analytics, 61% named delivering on data strategy as a top 3 priority, and 50% said they hoped to improve the data culture in their organizations. Among the key challenges they face is getting buy-in from the C-suite, with 71% indicating influencing the executive committee is a top 3 challenge. Rounding out the top 3 challenges are limited data skills (71%) and poor data literacy (54%).
Shifting performance metrics for analytics teams is also important. A greater focus on the impacts of insights rather than volume of reports could be one such shift.
There also needs to be an appetite for creativity and innovation among analytics teams. Building teams with different and diverse perspectives can lead to new ideas. Partnering with external expertise can also bring about fresh methodologies and insights to the table.
Finally, a data strategy roadmap must look for some evidence based quick wins along the implementation journey that demonstrate the effectiveness of change. This builds acceptance and motivation to move forward. It also becomes the foundation of evidence based change that permits for iterative adaption and pivots as required. In our experience this can be achieved with some simple micro controlled experiments before roll out is fully complete. This might be as simple as comparing application of analytics innovations to status quo processes. For example - how many more click throughs and conversions are achieved when leveraging lead scoring and segmented messaging over existing ‘shotgun’ approaches? Proving this is often when the penny drops.
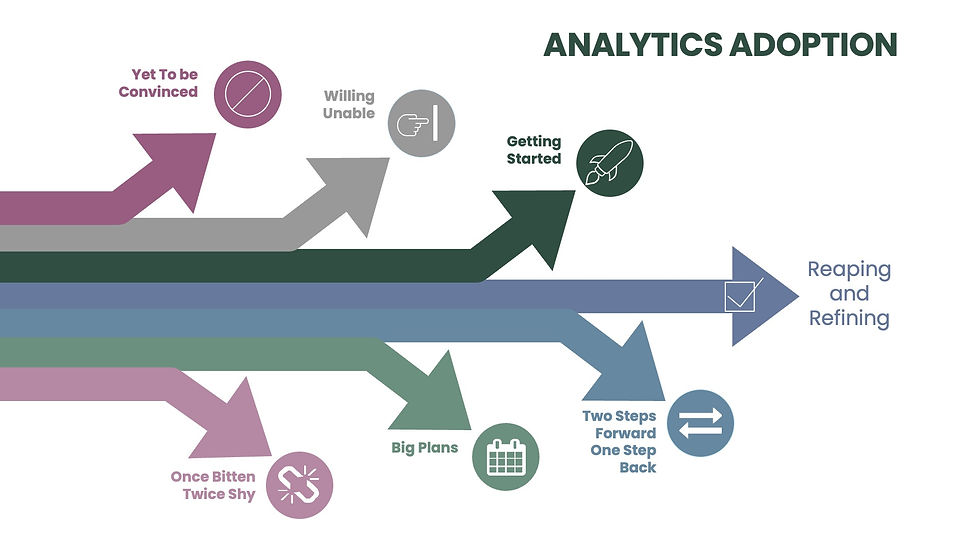
Humans meet the machine
Analytics mostly relies on tools and technology but ultimately, it’s the people behind the tools who will drive continuous improvement and innovation. While advances in generative AI, LLMs, machine learning and processing power all contribute to increasing capacity, they are, at least for the moment, still tools. Many organisations face pitfalls believing that flicking the switch on their newly acquired tech platforms will boost continuous improvement and innovation.
They still require people who can piece together the different tools and technology to provide the best business outcomes. As AI and tools continue to advance, lower-level analytics like BAU reports require less and less human interaction, freeing up the humans for higher order tasks and problem solving.
With exponential advances coming down the line, we all have to watch this space, but it’s reasonably safe to say that for now, the machines should focus on reducing the burden of our routine tasks - helping us (not replacing us) to identify the underlying patterns within complex data.
“In order to keep my pants up I have a belt… but it needs my pants’ belt holes to make it work…SO JUST WHO IS THE REAL HERO HERE?”- Mitch Hedberg
Final thoughts
Transitioning analytics from reporting to problem solving is a massive step but ultimately leads to innovation and more effective use of an organisation’s people, platforms or processes. The trick is to break this down into smaller steps that demonstrate wins along the way. Many mid-tier enterprises are realising they need to play catch up – this is no longer about surprising and delighting but is becoming a ticket to play. It’s time to empower analytics teams to transcend task and process orientation to realise their full potential as problem solvers and drivers of innovation.
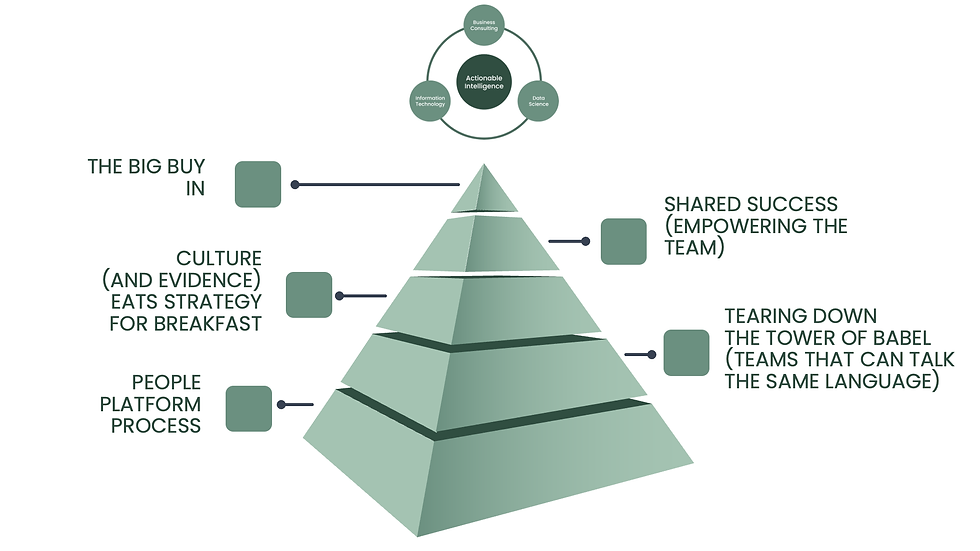
References
New Vantage Partners. Data and analytics leadership annual executive survey 2023. Wavestone. Accessed Februrary 2, 2024. https://www.wavestone.us/insights/data-and-analytics-leadership-annual-executive-survey-2023/
Gartner Hype cycle
https://www.gartner.com.au/en/methodologies/gartner-hype-cycle
2022-Deloitte-Chief-Data-Officer-Report.pdf
Five facts how customer-analytics boosts corporate performance
Author
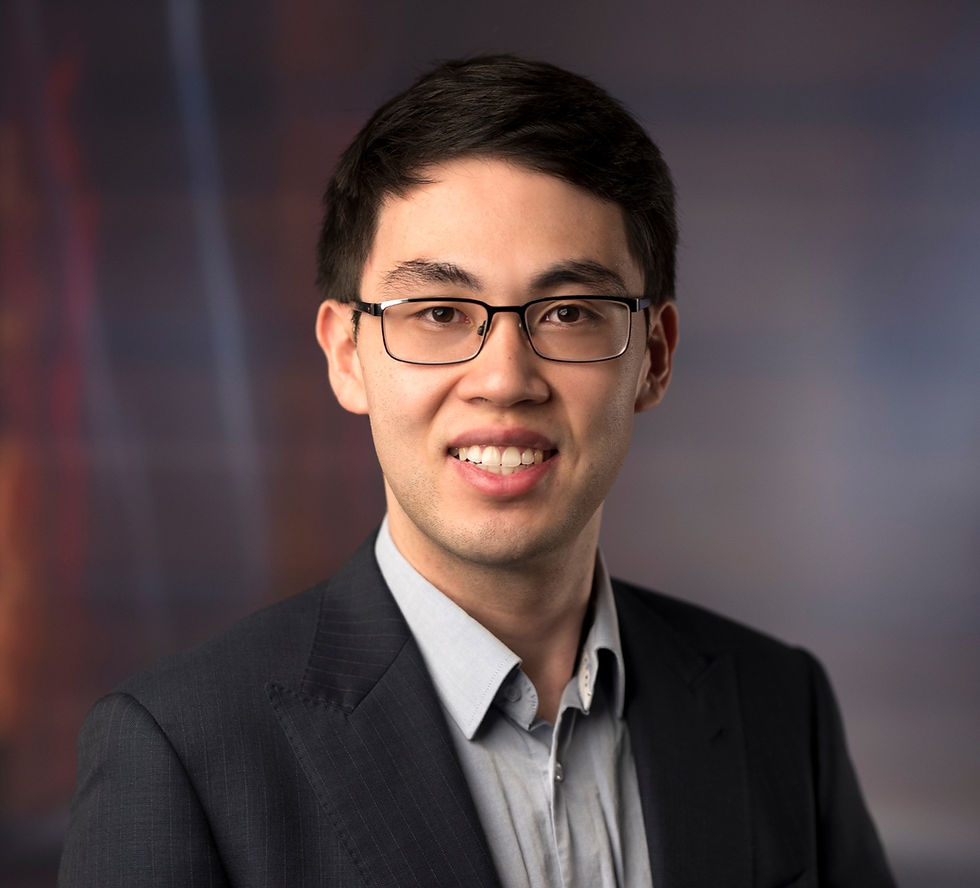
Anthony Zhang is a Senior Data Insights Consultant and analytics mavin. An experienced economic and social researcher, Anthony expertise lays in quantitative research and data science.
Comments